The function of a protein is often determined by how it folds into a 3D structure. Therefore, knowledge of a protein’s structure is essential for a deeper understanding of its role in various cellular processes. However, for most proteins known to mankind, our experimental knowledge lacks their determined structure. For instance, the universal protein database Uniprot archives 229 million unique protein sequences, while the Protein Data Bank, the single worldwide archive for experimentally resolved protein structures, holds 206,000 proteins. X-ray crystallography, or cryo-electron microscopy, the traditional protein-structure-determination method that fires X-rays or electron beams at proteins to create a picture of their shape, is very time-consuming and technologically challenging. It thus contributes to the massive (more than a 1,000-fold) gap between known protein sequences and experimental protein structures.
This gap could be closed by predicting proteins’ 3D configurations straight from their linear amino acid sequence, a solution that AlphaFold may offer. AlphaFold is a program powered by artificial intelligence (AI), developed by DeepMind, part of Alphabet Inc., Google’s parent company. AlphaFold transforms a protein’s sequence into its structure with high accuracy. EMBL-European Bioinformatics Institute (EMBL-EBI), partnering with DeepMind, made the predicted structures of over 200 million cataloged proteins available to science through the AlphaFold Protein Structure Database (AlphaFold DB). This freely available resource offers programmatic access to its data and interactive visualization of predicted structures.
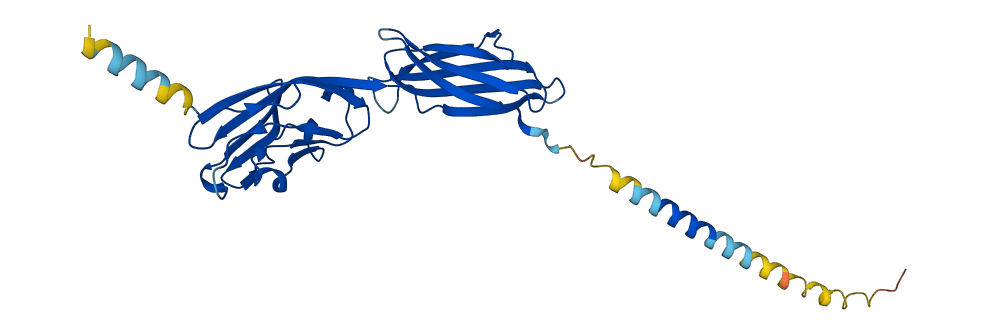
AlphaFold Data Copyright (2022) DeepMind Technologies Limited.
AlphaFold DB displays atomic coordinates and a per-residue confidence score (pLDDT), which are estimated for each predicted structure. pLDDT scores are on a scale from o to 100, with higher scores reflecting better confidence. Residues with pLDDT ≥ 90 have very high model confidence, while residues with 90 > pLDDT ≥ 70 are classified as confident. Residues with 70 > pLDDT ≥ 50 have low confidence, and residues with pLDDT < 50 correspond to very low confidence.
Access to the 3D shape of nearly all known proteins is a game changer in scientific research. The research community has widely embraced it. Since July 2021, with the release of a paper describing the AlphaFold software with the source code, along with the availability of AlphaFold DB, over 2,000 articles showcasing AlphaFold’s use have been published. Notable applications include fighting plastic pollution, gaining insights into Parkinson’s disease, and improving honey bees’ health.
While AlphaFold has revolutionized the field of structural biology, some limitations of this tool are worth noting. AlphaFold DB does not include structures for proteins that are longer than 2,700 residues. Around 207 large proteins with residue ranges from 2,701 to 34,350 are biologically important human proteins, such as those encoded by titin and dystrophin, but their full-length protein structures are unavailable in AlphaFold DB. Additionally, AlphaFold is designed to predict a single structure for a protein sequence. However, many proteins adopt multiple conformations, especially in the presence of interacting partners or molecules, such as ligands and drugs, which could be crucial to their function. Those multiple conformations are missing from AlphaFold DB.
To learn more about the AlphaFold database, please read the 2021 article about AlphaFold DB by Varadi et al. and the AlphaFold DB Frequently Asked Questions.
~Ansuman Chattopadhyay